~ 10 min read
DOI: https://doi.org/10.36850/4m4z-8784
Evaluative Measures or Game-Able Targets? Academic Competition Can Turn Even Qualitative Measures into Targets for Researchers
Following decades of increasingly precise measurement of researchers’ scientific output and achievements, the fashion of quantifying science is losing its shine. In China, for example, the amount of published papers and citations have lost their role as key indicators of a researcher’s success – despite an expected decrease in publication rate and university rankings [1, 2]. The German research council proposed similar far-reaching reforms in their science evaluation; they want their research system to attach less value to research quantity and pay more attention to other academic activities like teaching and giving policy advice [3]. The Dutch council, taking it one step further, is removing all references to impact factors and citations from their application forms, and has now put the ‘personal narrative’ at the heart of their public funding distribution [4, 5].
The reforms seem to come in due time. Measures like citation indexes, numbers of publications and university rankings are widely criticized because they are biased and fail to accurately measure the social impact and scientific relevance of research [6-9]. More importantly, these measures tend to slowly turn into goals on their own, and lead to questionable and fraudulent practices among researchers that help them increase their rankings by ‘gaming’ the evaluation system [10-15]. Will the new quality-focused evaluation measures serve their promise and bring these issues to a halt, or will they eventually turn into gameable targets as well? I would like to suggest that the latter option can only be prevented if the harsh competition for research funding and academic jobs cools down.
Bias and Goodhart's law
Quantification started to gain hold over scientific research during the late 20th century [16-18]. The overwhelming growth of scientific publications and institutions created a demand for indexes and rankings, which was consequently enabled by new methods from the social sciences and – at a later point – increasing digitalization. Growing amounts of public funding and socio-economic expectations of science lead governments to impose a strict management- and evaluation system that guaranteed high research output and global competitiveness of national science. Since then, not a single scientific institution or agent has been left unquantified. Publication- and citation rates have become the yardstick for measuring the output of individual researchers and gained a critical role in job applications [19]. Journals were (and still are) ranked by their Impact Factor, which is based upon the amount of high-citation articles they publish, and universities are classed by the amount of prizes and highly cited researchers they can muster.
The drawbacks of this trend became only apparent in recent two decades and can be divided into two categories. First, many of the standards used to evaluate science have turned out to be unfit for measuring scientific or social relevance. Measuring citations, for example, might give a rough overview of one’s impact in the field, but citation count can be biased against narrow sub-disciplines, multidisciplinary research, articles in non-English languages and early-career authors [6, 7]. In addition, citation count often overlooks the use and references of academic works in books, reviews, online discussions, public policy documents and public science communication [8]. The Journal Impact Factor is based on this citation count and therefore inherits the same biases and blind spots, which can lead to a skewed ranking of journals [9]. Furthermore, most measures of the scientific and social impact of research do not account for mentoring abilities, teaching, public communication and other academic activities that are hard to quantify.
The second category consists of issues that arise when the measures become targets in themselves, and then shape the researchers’ culture and practices accordingly. When this happens, the measures lose their evaluative power, an effect also known as ‘Goodhart’s Law’: “When a measure becomes a target, it ceases to be a good measure” [10]. The high value attributed to publication count has for instance been linked to the practice of ‘salami-slicing’, where researchers redundantly split up an article in multiple publications [11], and it incentivizes researchers to commit questionable research practices like p-value hacking, eliminating inconvenient data or even rigging the peer-review process [12]. The emphasis on citation as a golden evaluation standard has comparable flaws. It forces young researchers to limit themselves to popular fields, topics and methods, and places a high risk - like losing one’s job - on epistemic original and innovative work that might perhaps take time before being appreciated or recognized [13, 14]. In addition, highly valuing citation counts are associated with increased rates of self-citations and lengthy reference lists [10]. Self-citation is also seen in the journals, where editors recommend and even oblige authors to cite from self-owned journals in order to raise their Impact Factor [15].
To sum up, the measures used to evaluate science are not only mediocre in measuring social and scientific impact, they are also becoming less effective in doing so because researchers are treating them as targets.
Novel measures, new targets?
Since these flaws of quantification became known to scientific community, there has been an explosion of new measures, rankings and indexes that ought to solve some of these shortcomings [18]. The widely used h-index, for example, aims to get a more balanced view of researchers’ achievements by filtering out researchers with extremely popular papers and favouring researchers who consistently publish well-cited articles – but it still preserves practically all other flaws [8]. Another popular initiative is Altmetrics, a program that maps the societal impact of academic articles by tracking their presence on social media, news outlets and blogposts. However, measuring social impact in this manner might bias articles on topics that coincide with public interests, like the health benefits of alcohol or puppies, and might be easily gameable by creating or buying false likes and mentions [20].
Could reforms like the ones recently suggested by the Chinese, German and Dutch research councils offer a solution? By also including teaching, mentoring, social-economic impact and the use of academic work in public policy and communication, the scope of the impact measured could be broadened. In addition, new quality-focused measures, like time spend on teaching or the number of Master’s thesis’s guided, could be harder to game – although this is not necessarily the case. The Dutch research council’s shift to base their funding on narratives could for instance lead to a competition that favours good story writers and give rise to agencies that specialize in writing attractive narratives [21]. The new evaluation methods might measure impact more accurately but the danger of these measures turning into new targets remains a looming threat.
It might therefore be more fruitful to ask the question why gaming practices arise and become mainstream in the first place. Why and how do measures become targets? The different suggested solutions and reforms don’t offer an answer, but instead only propose us new evaluation methods. As an alternative, I suggest we should look at the incentive structure of science and its relation with the high competitiveness within academia [12, 22].
Competition and the selection of questionable practices
It is widely recognized that the competition for research funding and academic positions has increased in the last fifty years [22]. The growing supply of PhD graduates is not matched in terms of public funding or available research and teaching positions, which intensifies the competition for these resources [22, 23]. Many graduates will be filtered out and eventually have to leave academia, which results in a strong selective pressure on norms and practices that help them score high on whatever evaluative measures are currently in fashion. Researchers who use methods or hold beliefs and values that help them secure many citations, publications or satisfy other measures, will be less likely to drop out than researchers who attach more importance to ethical or epistemic considerations [24]. Subsequently, the researchers that survive this selection process will eventually become the teachers and role models of the next generation of students and graduates. Over time, this can gradually and unintentionally alter the academic culture and normalize gaming practices like self-citation and salami-slicing, or even popularize bad methods that are more likely to find false positives.
If these questionable practices are to be countered, research institutions should not only adopt new evaluation methods, but also investigate and decrease competition in academia. For sure, new measures focused on research quality could broaden the scope of the impact that is measured and perhaps even lower academic competition, but changing only the measures will not be sufficient. Only when competition is lowered by increasing career certainty, funding possibilities and raising researchers' intrinsic motivation, the questionable practices that treat evaluative measures as targets will lose their advantage in the struggle for academic existence.
References
[1]: Huang, F. (2020). China is choosing its own path on academic evaluation. University World News.
[2]: Mallapaty, S. (2020). China bans cash rewards for publishing papers. Nature, 579(7798), 18-19.
[3]: Boytchev, H. (2021). Science council calls for ‘system reboot’ after pandemic. Research Professional News.
[4]: Woolston, C. (2021). Impact factor abandoned by Dutch university in hiring and promotion decisions. Nature, 595(7867), 462-462.
[5]: NWO. (2022). Declaration on Research Assessment. Dutch Research Council. From: https://www.nwo.nl/en/dora
[6]: Kumar, M. J. (2009). Evaluating scientists: citations, impact factor, h-index, online page hits and what else?. IETE Technical Review, 26(3), 165-168.
[7]: Thwaites, T. (2014). Research metrics: Calling science to account. Nature, 511(7510), S57-S60.
[8]: Ravenscroft, J., Liakata, M., Clare, A., & Duma, D. (2017). Measuring scientific impact beyond academia: An assessment of existing impact metrics and proposed improvements. PloS one, 12(3), e0173152.
[9]: Neylon, C., & Wu, S. (2009). level metrics and the evolution of scientific impact. PLoS biology, 7(11), e1000242.
[10]: Fire, M., & Guestrin, C. (2019). Over-optimization of academic publishing metrics: observing Goodhart’s Law in action. GigaScience, 8(6), giz053.
[11]: Šupak Smolčić, V. (2013). Salami publication: Definitions and examples. Biochemia Medica, 23(3), 237-241.
[12]: Edwards, M. A., & Roy, S. (2017). Academic research in the 21st century: Maintaining scientific integrity in a climate of perverse incentives and hypercompetition. Environmental engineering science, 34(1), 51-61.
[13]: Müller, R., & Rijcke, S. D. (2017). Thinking with indicators. Exploring the Epistemic Impacts of Academic Performance Indicators in the Life Sciences. Correction to earlier article.
[14]: Pardo Guerra, J. P. (2020). Research metrics, labor markets, and epistemic change: evidence from Britain 1970-2018. SocArXiv. January, 28.
[15]: Wilhite, A. W., & Fong, E. A. (2012). Coercive citation in academic publishing. Science, 335(6068), 542-543.
[16]: Sugimoto, C. R., & Larivière, V. (2018). Measuring research: What everyone needs to know. Oxford University Press. 7-14.
[17]: Lane, J., & Bertuzzi, S. (2011). Measuring the results of science investments. Science, 331(6018), 678-680.
[18]: Van Noorden, R. (2010). A profusion of measures: scientific performance indicators are proliferating--leading researchers to ask afresh what they are measuring and why. Richard Van Noorden surveys the rapidly evolving ecosystem. Nature, 465(7300), 864-867.
[19]: Petersen, A. M., Wang, F. & Stanley, H. E. (2010). Methods for measuring the citations and productivity of scientists across time and discipline. Physical Review E, 81(3), 036114.
[20]: Bornmann L. (2014) Do altmetrics point to the broader impact of research? An overview of benefits and disadvantages of altmetrics. Journal of Informetrics. 2014;8(4):895–903.
[21]: Bronkhorst, X. (2022). Hoogleraar Hans Clevers waarschuwt voor “desastreuze” gevolven Erkennen & Waarderen. Digital University Magazine DUB.
[22]: Anderson, M. S., Ronning, E. A., De Vries, R., & Martinson, B. C. (2007). The perverse effects of competition on scientists’ work and relationships. Science and engineering ethics, 13(4), 437-461.
[23]: Rathenau Institute. (2021). Aanvraagdruk bij NWO, from: https://www.rathenau.nl/nl/wetenschap-cijfers/werking-van-de-wetenschap/excellentie/aanvraagdruk-bij-nwo
[24]: Smaldino, P. E., & McElreath, R. (2016). The natural selection of bad science. Royal Society open science, 3(9), 160384.
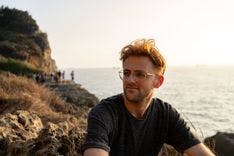
Marcel Hobma is a student in History and Philosophy of Science, and trained as an (investigative) journalist. His interests vary from the Philosophy of Biology to the incentive structure of science. He currently works on his Master's thesis on the cultural evolution of values in nutrition science.